The challenges with building an AI product with transparency at its core


In an era where AI-driven products are rapidly revolutionizing the world, we continue to witness the emergence of new AI models every week. AI startups encounter considerable challenges in gaining user adoption for their new product features that leverage advanced AI models, even when these models may offer superior performance. One of the fundamental challenges is a lack of user trust in AI. This skepticism isn't baseless but rooted in the perceived opacity of AI operations. To overcome this, adopting transparency in the development and operation of AI products is fundamental.
What are challenges in launching an AI product?
AI products, as fascinating as they are, pose some significant transparency challenges:
Users are often left in the dark about what specific AI model is being used. Understanding the AI model's function is critical for trust-building, but this lack of disclosure leaves a knowledge gap.Â
User concerns arise about what kind of user data is used as input. Users have a right to know what personal data is collected and utilized by these systems.Â
The opacity about how this data traverses the interconnected systems adds to the mistrust. Without clarity on data handling procedures, users (and enteprises adopting AI tools) can feel uneasy about potential data leakages.
The storage of data is another grey area. Users remain largely unaware of where their information is stored and how securely it is kept. Additionally, when data residency is a requirement for certain customers, the storage question can have significant legal ramifications if not managed in line with information security policies.
Ambiguity surrounding data retention duration compounds these issues. Without clear durations on data storage, questions about perpetual data usage necessarily arise - thereby exacerbating user concerns.
How can these challenges be addressed?
Let's delve into a common AI product architecture where a user connects their own data from tools (eg: Notion, slack, Teams ect) that they use to do their work, and explore potential solutions to tackle these challenges.
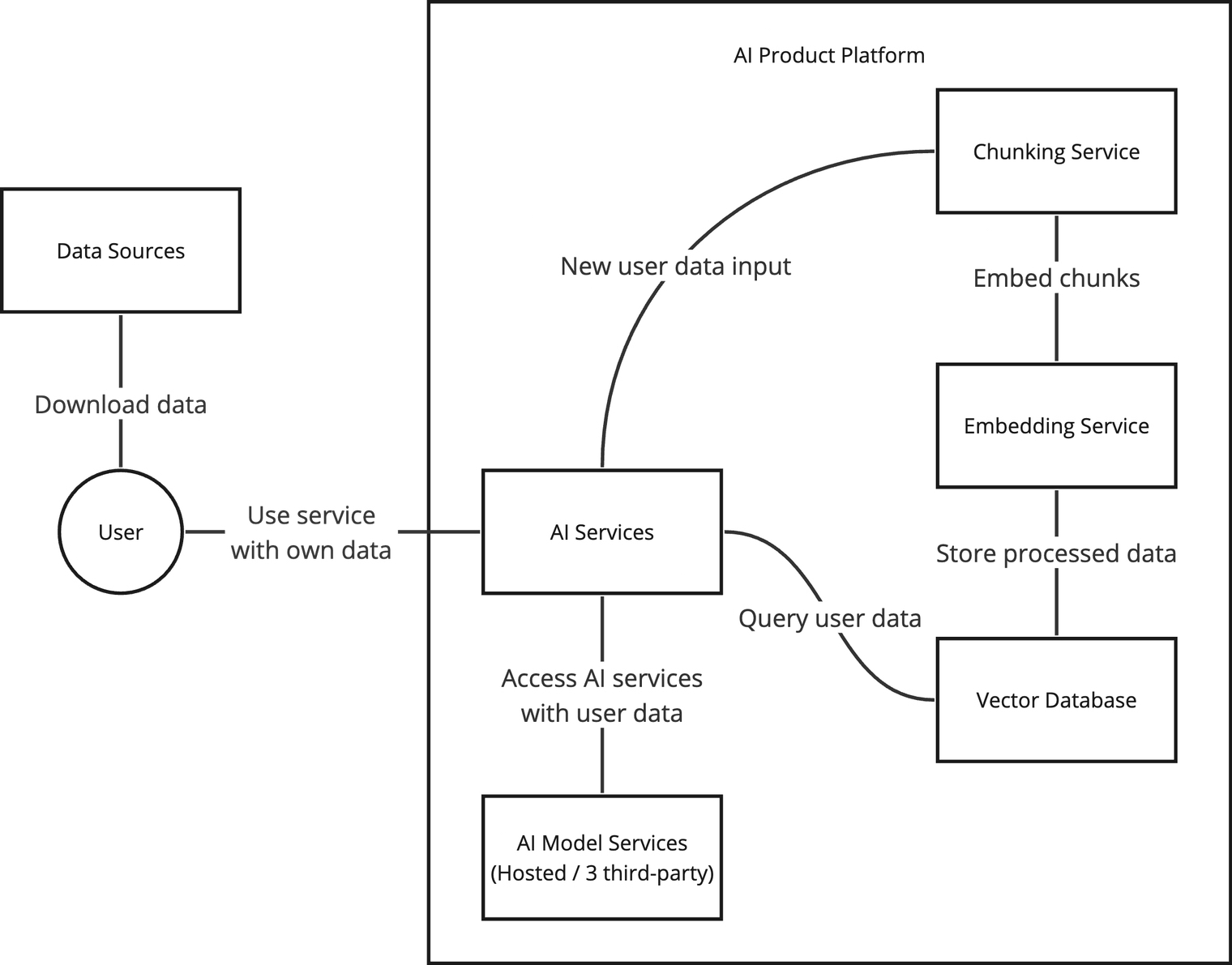
Architecture Overview for typical AI Product
One solution is to integrate user activity logging to establish a transparent data processing pipeline. Actions carried out by AI platform services are logged, providing an interface to allow users to view what is happening after they upload their data. Additionally, you can record the embedding model used and the AI inference model applied.
Secondly, you can introduce a data governance service. This service will actively monitor the database, ensuring effective data management without compromising production stability. Here it can prompt users to review data source being used when necessary, enhancing awareness and accountability in data usage.
What are the costs of implementing the transparency process?
Time cost
To begin with, the process can be notably time-consuming, tasks such as developing user interfaces, establishing clear communication channels for data usage and transfer, and creating robust AI governance processes require substantial time and effort.
More resources
Effectively implementing these transparency measures also demands extra resources. This includes dedicated personnel for development and governance, as well as additional hardware and software resources. While these supplementary costs can be daunting, especially for start-ups, they represent crucial investments in building an AI product that users can trust.
Opportunity cost
These efforts may inherently result in a slowdown of product feature releases. Incorporating transparency into various aspects of the AI product life cycle involves additional steps that can extend the timeline, potentially causing delays in the introduction of new features.
We can help with that
The issue of user distrust is primarily rooted in the opaque nature of AI operations, underscoring the importance of transparency. Building trust with users involves implementing features that allow them to observe how AI products interact with data and exercise control over the data consumed by AI services. At Redactive, our focus lies in addressing these aspects, and we are well-equipped to assist you in navigating swiftly through dynamic environments.
We are actively engaged in refining a data governance framework to elevate the evolution of AI products. Our solution brings forth a secure real-time data access model, streamlining the data ingestion process while ensuring robust protection for data querying. Instead of store the user data, we index the user data for more secure data query model & are delivering an activity logging system to keep check of how data is being accessed across the data pipeline, from data source to end user access.
With Redactive, AI product builders can focus on shaping the fundamental product features, relying on our integration of AI governance intertwined with a secure data access model. Here, builders don’t need to concern themselves with the development of data source integrations.
Forget about chunking, embedding and Vector Databases. Redactive's managed RAG pipelines make it easy to access the context you need via our SDK.